Our team have been offering SEO expert services to clients since the early days of the internet. Whilst the tactics have evolved over the years, our overall goal by no means has and thats making sure our users web pages to rank on page 1 for appropriate keywords at the same time only using honest and long term strategies.
Tuesday, January 31, 2017
The Essential Artificial Intelligence Glossary for Marketers
Thank goodness for live chat. If you’re anything like me, you look back at the days of corded phones and 1-800 numbers with anything but fondness.
But as you’re chatting with a customer service agent on Facebook Messenger to see if you can change the shipping address on your recent order, sometimes it’s tempting to ask, am I really talking to a human? Or is this kind, speedy agent really just a robot in disguise?
Believe it or not, this question is older than you might think. The game of trying to decipher between human and machine goes all the way back to 1950 and a computer scientist named Alan Turing.
In his famous paper, Turing proposed a test (now referred to as the Turing Test) to see if a machine’s ability to exhibit intelligent behavior is indistinguishable from that of a human. An interrogator would ask text-based questions to subject A (a computer) and subject B (a person), in hopes of trying to figure out which was which. If the computer successfully fooled the interrogator into thinking it was a human, the computer was said to successfully have artificial intelligence.
Since the days of Alan Turing, there’s been decades and decades of debate on if his test really is an accurate method for identifying artificial intelligence. However, the sentiment behind the idea remains: As AI gains traction, will we be able to tell the difference between human and machine? And if AI is already transforming the way we want customer service, how else could it change our jobs as marketers?
Why Artificial Intelligence Matters for Marketers
As Turing predicted, the concepts behind AI are often hard to grasp, and sometimes even more difficult recognize in our daily lives. By its very nature, AI is designed to flow seamlessly into the tools you already use to make the tasks you do more accurate or efficient. For example, if you’ve enjoyed Netflix movie suggestions or Spotify’s personalized playlists, you’re already encountering AI.
In fact, in our recent HubSpot Research Report on the adoption of artificial intelligence, we found that 63% of respondents are already using AI without realizing it.
When it comes to marketing, AI is positioned to change nearly every part of marketing -- from our personal productivity to our business’s operations -- over the next few years. Imagine having a to-do list automatically prioritized based on your work habits, or your content personalized based on your target customer writes on social media. These examples are just the beginning of how AI will affect the way marketers work.
No matter how much AI changes our job, we’re not all called to be expert computer scientists. However, it’s still crucial to have a basic understanding how AI works, if only to get a glimpse of the possibilities with this type of technology and to see how it could make you a more efficient, more data-driven marketer.
Below we’ll break down the key terms you’ll need to know to be a successful marketer in an AI world. But first, a disclaimer ...
This isn’t meant to be the ultimate resource of artificial intelligence by any means, nor should any 1,500-word blog post. There remains a lot of disagreement around what people consider AI to be and what it’s not. But we do hope these basic definitions will make AI and its related concepts a little easier to grasp and excite you to learn more about the future of marketing.
13 Artificial Intelligence Terms Marketers Need to Know
Algorithm
An algorithm is a formula that represents the relationship between variables. Social media marketers are likely familiar, as Facebook, Twitter, and Instagram all use algorithms to determine what posts you see in a news feed. SEO marketers focus specifically on search engine algorithms to get their content ranking on the first page of search results. Even your Netflix home page uses an algorithm to suggest new shows based on past behavior.
When you’re talking about artificial intelligence, algorithms are what machine learning programs use to make predictions from the data sets they analyze. For example, if a machine learning program were to analyze the performance of a bunch of Facebook posts, it could create an algorithm to determine which blog titles get the most clicks for future posts.
Artificial Intelligence
In the most general of terms, artificial intelligence refers to an area of computer science that makes machines do things that would require intelligence if done by a human. This includes tasks such as learning, seeing, talking, socializing, reasoning, or problem solving.
However, it’s not as simple as copying the way the human brain works, neuron by neuron. It’s building flexible computers that can take creative actions that maximize their chances of success to a specific goal.
Bots
Bots (also known as “chatbots” or “chatterbots”) are text-based programs that humans communicate with to automate specific actions or seek information. Generally, they “live” inside of another messaging application, such as Slack, Facebook Messenger, WhatsApp, or Line.
Bots often have a narrow use case because they are programmed to pull from a specific data source, such as a bot that tells you the weather or helps you register to vote. In some cases, they are able to integrate with systems you already use to increase productivity. For example, GrowthBot -- a bot for marketing and sales professionals -- connects with HubSpot, Google Analytics, and more to deliver information on a company’s top-viewed blog post or the PPC keywords a competitor is buying.
Some argue that chatbots don’t qualify as AI because they rely heavily on pre-loaded responses or actions and can’t “think” for themselves. However, others see bots’ ability to understand human language as a basic application of AI.
Cognitive Science
Zoom out from artificial intelligence and you’ve got cognitive science. It’s the interdisciplinary study of the mind and its processes, pulling from the foundations of philosophy, psychology, linguistics, anthropology, and neuroscience.
Artificial intelligence is just one application of cognitive science that looks at how the systems of the mind can be simulated in machines.
Computer Vision
Computer vision is an application of deep learning (see below) that can “understand” digital images.
For humans, of course, understanding images is one of our more basic functions. You see a ball thrown at you and you catch it. But for a computer to see an image and then describe it makes simulating the way the human eye and brain work together pretty complicated. For example, imagine how a self-driving car would need to recognize and respond to stop lights, pedestrians, and other obstructions to be allowed on the road.
However, you don’t have to own a Tesla to experience computer vision. You can put Google’s Quick Draw to the test and see if it recognizes your doodles. Because computer vision uses machine learning that improves over time, you’ll actually help teach the program just by playing.
Data Mining
Data mining is the process of computers discovering patterns within large data sets. For example, an ecommerce company like Amazon could use data mining to analyze customer data and give product suggestions through the “customers who bought this item also bought” box.
Deep Learning
On the far end of the AI spectrum, deep learning is a highly advanced subset of machine learning. It’s unlikely you’ll need to understand the inner workings of deep learning, but know this: Deep learning can find super complex patterns in data sets by using multiple layers of correlations. In the simplest of terms, it does this by mimicking the way neurons are layered in your own brain. That’s why computer scientists refer to this type of machine learning as a “neural network.”
Machine Learning
Of all the subdisciplines of AI, some of the most exciting advances have been made within machine learning. In short, machine learning is the ability for a program to absorb huge amounts of data and create predictive algorithms.
If you’ve ever heard that AI allows computers to learn over time, you were likely learning about machine learning. Programs with machine learning discover patterns in data sets that help them achieve a goal. As they analyze more data, they adjust their behavior to reach their goal more efficiently.
That data could be anything: a marketing software full of email open rates or a database of baseball batting averages. Because machine learning gives computers to learn without being explicitly programmed (like most bots), they are often described as being able to learn like a young child does: by experience.
Natural Language Processing
Natural language processing (NLS) can make bots a bit more sophisticated by enabling them to understand text or voice commands. For example, when you talk to Siri, she’s transposing your voice into text, conducting the query via a search engine, and responding back in human syntax.
On a basic level, spell check in a Word document or translation services on Google are both examples of NLS. More advanced applications of NLS can learn to pick up on humor or emotion.
Semantic Analysis
Semantic analysis is, first and foremost, a linguistics term that deals with process of stringing together phrases, clauses, sentences, and paragraphs into coherent writing. But it also refers to building language in the context of culture.
So, if a machine that has natural language processing capabilities can also use semantic analysis, that likely means it can understand human language and pick up on the contextual cues needed to understand idioms, metaphors, and other figures of speech. As AI-powered marketing applications advance in areas like content automation, you can imagine the usefulness of semantic analysis to craft blog posts and ebooks that are indistinguishable than that of a content marketer.
Supervised Learning
Supervised learning is a type of machine learning in which humans input specific data sets and supervise much of the process, hence the name. In supervised learning, the sample data is labeled and the machine learning program is given a clear outcome to work toward.
Training Data
In machine learning, the training data is the data initially given to the program to “learn” and identify patterns. Afterwards, more test data sets are given to the machine learning program to check the patterns for accuracy.
Unsupervised Learning
Unsupervised learning is another type of machine learning that uses very little to no human involvement. The machine learning program is left to find patterns and draw conclusions on its own.
Have an artificial intelligence definition to add? Let us know in the comment below.
Why Design and Coding Academies Need to Get in on Inbound Marketing
Supermodels may have ruled the world in the 1990s, but today it's the creatives. Everyone wants in on disruptive, viral, [insert your own buzz word here] intersecting worlds of design and technology. Especially the savvy students who are looking for challenging, fun, and economically rewarding professions.
Design and coding academies are rising to meet these opportunities, but there's a lot of noise swirling around. If you want your academy's message to shout out and reach your prospective students, you need to be using inbound and content marketing.
Fortunately, your creative natures and enterprises are great fits to achieve great results with content marketing and inbound.
You're Already Flush with Content
For a lot of marketing teams, creating quality content on a consistent basis is a big challenge. Not so for design and coding academies. Your faculty and students already create amazing content daily. Student projects, faculty lectures, and documented curricula are all content sources ready to be tapped.
You can video individual lectures from different courses and post clips online. You're naturals for developing some of the best visual content out there. Take some screenshots of the design or coding tools you teach students to use and add some eye-popping captions as a mini-tutorial. Share your faculty's expertise by publishing their work paired with a short back story or interview with that teacher.
Getting creative is your jam. Review the wealth of content your community is already creating through the lens of your content strategy to attract new students. You'll see your opportunities.
Improve Your SEO by Repurposing Your Content
The first step with the inbound methodology is attracting your target audience. This requires an SEO strategy based on relevant keywords and topics. A blog talking about things your personas don't care about isn't going to help your academy get found.
After you've done some SEO research, freshen up blog posts, newsletter articles, and other content you already have. Let's say your research tells you that prospective students are curious about mobile UX design best practices. Now you can add new a summary, keywords and tags to a lecture video or presentation you've posted on this topic that are more relevant. Instead of captioning it "Introductory Lecture on Mobile Design," you can change it to "Mobile UX Design: Learning Best Practices for Mobile Apps" (or whatever your research indicates).
Understanding what your SEO research is telling you will also help you select the most useful and on-point content to repurpose. Your academy does have a wealth of content, but that doesn't mean you want to throw all of it up to see what sticks. You want to make strategic selections of what content to look for, what to create, and how to optimize it for SEO so you make best use of your resources.
Your Content Will Build Your Reputation
The linchpin of finding success with inbound marketing is using your content to build trust with your target personas. Academies and bootcamps don't have the brand recognition that traditional schools enjoy. The waters are also muddied by the explosion in your direct competition.
The number of coding academies grew by nearly 50% in 2016 and is projected to continue growing rapidly. If you want to carve out a spot on the leader board, then you need to boost your brand recognition and make sure your brand connotes credibility and authority.
Prospective students won't trust putting their professional training in your hands if they don't see you as a go-to source on topics related to coding or design. Use your content to build up their confidence that your academy is at the front edge of your field and has the chops to make them employer-magnets after you graduate them.
You can share recent alumni stories that show how easily your graduates transition from students into lucrative careers. Quintupling your salary post-graduation? That's not a bad haul.
Build your reputation as masters of your field by regularly publishing content that addresses its most pressing issues and trends. Publish your academy's own insights on everything from your field's fundamentals to controversial issues. The phrase is cliché, but you need to become a thought leader, not just as an academic institution, but within the professions you're training students to enter.
Your prospective students are out there, dying for clear guidance and answers to their most pressing questions. If you can be resource that answers them, you'll be the academy they choose.
Why 2017 Is the Year to Take Snapchat Seriously [Infographic]
Here at HubSpot, we're not shy about our fondness of Snapchat. Heck, we even devoted an entire day to recruiting via Snapchat. But maybe not everyone is as crazy about the app as we are. Personal feelings aside, it's time to start taking it seriously.
If nothing else, there's something to be said for Snapchat's off-the-charts growth. It's shown a 12% average year-over-year increase in revenue since 2014, and is estimated to earn just short of $1 billion is 2017. Why is it worth so much? Because people are listening and watching. In fact, content posted to the app gets, in total, 10 million views every day.
So, are you ready to start listening and watching, too? If you're not on board with Snapchat yet, have a look at this helpful infographic from our friends at WebpageFX. Think about what these figures might mean to your own organization -- and start snapping.

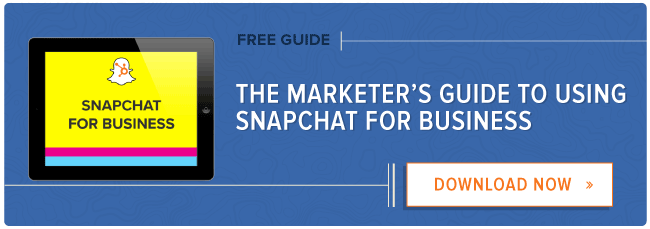
104 Email Marketing Myths, Experiments & Inspiring Tips [Free Guide]
As the number of email senders fighting for recipients’ attention continues to climb, many marketers are seeing their engagement rates steadily decrease -- even if they're using an approach that worked well a year or two ago. But that’s the problem: If you haven’t shaken up your email program in over a year, your emails are probably getting stale.
Whether you’re an email marketing veteran or are just getting started, you may be operating under certain common misconceptions about email that have been disproved by research. Even worse, your email may be getting dull due to a lack of experimentation or inspiration. Maybe you’re aware that it’s time to switch up your email marketing, but it can still be hard to know where to begin.
HubSpot and SendGrid are here to help. We’ve joined forces to jumpstart your effort to redefine your email program. In doing so, we created a guide with over 100 rigorously-researched tips on how to avoid common misconceptions, figure out what works for your audience, and bring new inspiration to your email program. Inside, you’ll find:
- Facts to help you push past the newest and most stubborn email marketing myths
- Test and experiment ideas you can use to optimize your email campaigns
- Tips and inspiration to help you identify ways to keep your emails fresh and engaging
- Extra juicy pro tips you can share with your network with one click
Click here to download 104 Email Marketing Myths, Experiments, and Inspiration
How Your Agency Can Use Social Media to Attract New Talent
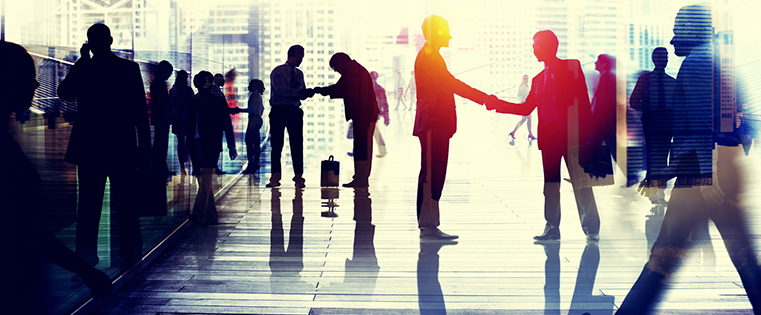
In 2015, GE ran an amusing ad campaign featuring Owen, a newly hired programmer trying to explain to family and friends why they should be excited about his new job. They were baffled that a programmer would work at what they believed was just a manufacturing company, offering only condolences as he tried to outline the innovative nature of a more modern GE.
The spot was a clever way for GE to spread the word about its position as a digital industrial company, and not just a manufacturing one. Simultaneously, it helped boost the recruiting of young, tech-savvy developers into the fold. After the ad campaign aired, GE’s online recruitment site received 66 percent more visits month after month.
With the exception of GE -- whose goal was to let people know that it does more than manufacture -- the top agencies often offer similar services with little or no variation. Beyond that, what separates the greats is their personalities: their people, their values, their approach to business, and their interactions with their communities.
In today's culture, who you work with is becoming more important than who you work for, and digital marketing is the best way to show prospective employees who you are as an agency, from the bottom up. By aligning digital marketing with HR, marketing agencies have the potential to create a sense of community that draws potential employees, clients, and market influencers closer than ever before.
Blurring the Lines Between HR and Marketing
Before the "Owen" ads brought more attention to GE, recent trends among other companies were already blurring the lines between HR and digital marketing. For instance, leaders are recognizing that employees are the best influencers, and have started leveraging them on social media channels, where employees can share company posts with their networks.
Certain corporate campuses even have designated areas for Instagram moments, allowing people to take photos with specific corporate campus attributes in the backdrop. The trend has spread all the way to the top, with CEOs like Meg Whitman posting pictures of their workspaces to promote more transparency.
By aligning HR's and marketing's methods and goals, you place your agency's HR personnel in a more strategic position to achieve their recruiting goals. It also forces the creation of a single, consistent brand that accurately portrays your agency's values and community.
Using Social Media to Attract New Employees
Most consumers today wouldn't make a large purchase without first researching the brand. Likewise, practical job candidates will likely research multiple agencies’ brands before applying or accepting a job at any of them. In fact, company websites are a top resource for candidates on the job hunt. Keep your agency's online representation consistent -- and therefore more attractive to interested parties -- with these five tips:
1) Highlight your agency's culture on social media.
Every social media platform caters to a different need, and understanding the gains of each will help you use social media to its utmost potential. For instance, use Snapchat to tell quick visual stories that showcase the agency's inner culture. Use Instagram to share photos of daily office life, and Twitter to showcase your agency's unique personality and content.
If your agency doesn't have a strong social media presence in 2017, you not only limit your chances of getting discovered by potential job seekers, you also risk turning off candidates in the process of researching your agency. Job seekers want to be able to get a good sense of what working for your agency is like on a daily basis. Without sharing content on social media that highlights this, they could get the impression that you don't value transparency.
2) Build employee advocacy.
In addition to using your agency's social media presence to showcase your teams's culture, it's important to build up employee advocacy. Social media isn't going away, and empowering employees to use it to the agency's advantage isn't difficult.
Encourage employees to share their own insights about working for your agency on LinkedIn, Facebook, Twitter, Instagram, and other relevant social media platforms. Their extended networks will see their posts and spread awareness of your agency's brand. By leveraging your employee's personal and professional networks, your agency has the potential to reach people you wouldn't have otherwise been able to with your business presence alone.
3) Connect with influencers in your agency's niche.
Digital marketing also allows your recruiting team to more easily interact with potential candidates, as well as the most influential individuals and companies in your market. Like them on Facebook, interact with their tweets, and follow the trends that research shows are proving the most successful.
4) Network freely.
Networking is at the heart of social media and digital marketing, so use media extensively to connect and network with potential candidates, clients, and companies that you work with. Capital One, for example, offers another idea of how increased networking boosted HR performance. Just this year, Capital One’s CIO attended the Grace Hopper Celebration of Women in Technology, specifically promoting Capital One’s digital products and, most importantly, its company culture.
5) Aim to amplify your message.
Connecting and networking with the right influencers also gives you the opportunity to amplify your message to a significantly larger audience. If you build employee advocacy, utilize social platforms to your advantage, and master the art of networking, you’ll see your brand spread exponentially every time someone posts, tweets, or Snapchats something about you. Others will see it, too, including the hundreds of thousands of followers your connections will be able to reach.
When employees share content about their company, those shares receive up to eight times more engagement and are reshared up to 25 times more frequently than the content on the brand’s page. Research shows that such strong engagement and company culture helps companies outperform their peers in "profitability, productivity, customer satisfaction, employee turnover,”"and more.
And by participating in social media and mobile apps, companies like Target have been able to attract and retain top talent while demonstrating its success with digital marketing. Because its digital marketing efforts are so extensive, those seeking new jobs already have an idea of what Target’s initiatives are.
GE’s engagement campaign, along with those of many other companies, offer a glimpse into how focusing more on value and results can boost an agency's image, performance, and recruitment efforts across the board. Follow their example, introduce your agency's personality to the world, and let top-level talent know who you are and how well they would fit into your agency's culture.
Google Search Console Reliability: Webmaster Tools on Trial
Posted by rjonesx.
There are a handful of data sources relied upon by nearly every search engine optimizer. Google Search Console (formerly Google Webmaster Tools) has perhaps become the most ubiquitous. There are simply some things you can do with GSC, like disavowing links, that cannot be accomplished anywhere else, so we are in some ways forced to rely upon it. But, like all sources of knowledge, we must put it to the test to determine its trustworthiness — can we stake our craft on its recommendations? Let's see if we can pull back the curtain on GSC data and determine, once and for all, how skeptical we should be of the data it provides.
Testing data sources
Before we dive in, I think it is worth having a quick discussion about how we might address this problem. There are basically two concepts that I want to introduce for the sake of this analysis: internal validity and external validity.
Internal validity refers to whether the data accurately represents what Google knows about your site.
External validity refers to whether the data accurately represents the web.
These two concepts are extremely important for our discussion. Depending upon the problem we are addressing as SEOs, we may care more about one or another. For example, let's assume that page speed was an incredibly important ranking factor and we wanted to help a customer. We would likely be concerned with the internal validity of GSC's "time spent downloading a page" metric because, regardless of what happens to a real user, if Google thinks the page is slow, we will lose rankings. We would rely on this metric insofar as we were confident it represented what Google believes about the customer's site. On the other hand, if we are trying to prevent Google from finding bad links, we would be concerned about the external validity of the "links to your site" section because, while Google might already know about some bad links, we want to make sure there aren't any others that Google could stumble upon. Thus, depending on how well GSC's sample links comprehensively describe the links across the web, we might reject that metric and use a combination of other sources (like Open Site Explorer, Majestic, and Ahrefs) which will give us greater coverage.
The point of this exercise is simply to say that we can judge GSC's data from multiple perspectives, and it is important to tease these out so we know when it is reasonable to rely upon GSC.
GSC Section 1: HTML Improvements
Of the many useful features in GSC, Google provides a list of some common HTML errors it discovered in the course of crawling your site. This section, located at Search Appearance > HTML Improvements, lists off several potential errors including Duplicate Titles, Duplicate Descriptions, and other actionable recommendations. Fortunately, this first example gives us an opportunity to outline methods for testing both the internal and external validity of the data. As you can see in the screenshot below, GSC has found duplicate meta descriptions because a website has case insensitive URLs and no canonical tag or redirect to fix it. Essentially, you can reach the page from either /Page.aspx or /page.aspx, and this is apparent as Googlebot had found the URL both with and without capitalization. Let's test Google's recommendation to see if it is externally and internally valid.
External Validity: In this case, the external validity is simply whether the data accurately reflects pages as they appear on the Internet. As one can imagine, the list of HTML improvements can be woefully out of date dependent upon the crawl rate of your site. In this case, the site had previously repaired the issue with a 301 redirect.
This really isn't terribly surprising. Google shouldn't be expected to update this section of GSC every time you apply a correction to your website. However, it does illustrate a common problem with GSC. Many of the issues GSC alerts you to may have already been fixed by you or your web developer. I don't think this is a fault with GSC by any stretch of the imagination, just a limitation that can only be addressed by more frequent, deliberate crawls like Moz Pro's Crawl Audit or a standalone tool like Screaming Frog.
Internal Validity: This is where things start to get interesting. While it is unsurprising that Google doesn't crawl your site so frequently as to capture updates to your site in real-time, it is reasonable to expect that what Google has crawled would be reflected accurately in GSC. This doesn't appear to be the case.
By executing an info:http://concerning-url query in Google with upper-case letters, we can determine some information about what Google knows about the URL. Google returns results for the lower-case version of the URL! This indicates that Google both knows about the 301 redirect correcting the problem and has corrected it in their search index. As you can imagine, this presents us with quite a problem. HTML Improvement recommendations in GSC not only may not reflect changes you made to your site, it might not even reflect corrections Google is already aware of. Given this difference, it almost always makes sense to crawl your site for these types of issues in addition to using GSC.
GSC Section 2: Index Status
The next metric we are going to tackle is Google's Index Status, which is supposed to provide you with an accurate number of pages Google has indexed from your site. This section is located at Google Index > Index Status. This particular metric can only be tested for internal validity since it is specifically providing us with information about Google itself. There are a couple of ways we could address this...
- We could compare the number provided in GSC to site: commands
- We could compare the number provided in GSC to the number of internal links to the homepage in the internal links section (assuming 1 link to homepage from every page on the site)
We opted for both. The biggest problem with this particular metric is being certain what it is measuring. Because GSC allows you to authorize the http, https, www, and non-www version of your site independently, it can be confusing as to what is included in the Index Status metric.
We found that when carefully applied to ensure no crossover of varying types (https vs http, www vs non-www), the Index Status metric seemed to be quite well correlated with the site:site.com query in Google, especially on smaller sites. The larger the site, the more fluctuation we saw in these numbers, but this could be accounted for by approximations performed by the site: command.
We found the link count method to be difficult to use, though. Consider the graphic above. The site in question has 1,587 pages indexed according to GSC, but the home page to that site has 7,080 internal links. This seems highly unrealistic, as we were unable to find a single page, much less the majority of pages, with 4 or more links back to the home page. However, given the consistency with the site: command and GSC's Index Status, I believe this is more of a problem with the way internal links are represented than with the Index Status metric.
I think it is safe to conclude that the Index Status metric is probably the most reliable one available to us in regards to the number of pages actually included in Google's index.
GSC Section 3: Internal Links
The Internal Links section found under Search Traffic > Internal Links seems to be rarely used, but can be quite insightful. If External Links tells Google what others think is important on your site, then Internal Links tell Google what you think is important on your site. This section once again serves as a useful example of knowing the difference between what Google believes about your site and what is actually true of your site.
Testing this metric was fairly straightforward. We took the internal links numbers provided by GSC and compared them to full site crawls. We could then determine whether Google's crawl was fairly representative of the actual site.
Generally speaking, the two were modestly correlated with some fairly significant deviation. As an SEO, I find this incredibly important. Google does not start at your home page and crawl your site in the same way that your standard site crawlers do (like the one included in Moz Pro). Googlebot approaches your site via a combination of external links, internal links, sitemaps, redirects, etc. that can give a very different picture. In fact, we found several examples where a full site crawl unearthed hundreds of internal links that Googlebot had missed. Navigational pages, like category pages in the blog, were crawled less frequently, so certain pages didn't accumulate nearly as many links in GSC as one would have expected having looked only at a traditional crawl.
As search marketers, in this case we must be concerned with internal validity, or what Google believes about our site. I highly recommend comparing Google's numbers to your own site crawl to determine if there is important content which Google determines you have ignored in your internal linking.
GSC Section 4: Links to Your Site
Link data is always one of the most sought-after metrics in our industry, and rightly so. External links continue to be the strongest predictive factor for rankings and Google has admitted as much time and time again. So how does GSC's link data measure up?
In this analysis, we compared the links presented to us by GSC to those presented by Ahrefs, Majestic, and Moz for whether those links are still live. To be fair to GSC, which provides only a sampling of links, we only used sites that had fewer than 1,000 total backlinks, increasing the likelihood that we get a full picture (or at least close to it) from GSC. The results are startling. GSC's lists, both "sample links" and "latest links," were the lowest-performing in terms of "live links" for every site we tested, never once beating out Moz, Majestic, or Ahrefs.
I do want to be clear and upfront about Moz's performance in this particular test. Because Moz has a smaller total index, it is likely we only surface higher-quality, long-lasting links. Our out-performing Majestic and Ahrefs by just a couple of percentage points is likely a side effect of index size and not reflective of a substantial difference. However, the several percentage points which separate GSC from all 3 link indexes cannot be ignored. In terms of external validity — that is to say, how well this data reflects what is actually happening on the web — GSC is out-performed by third-party indexes.
But what about internal validity? Does GSC give us a fresh look at Google's actual backlink index? It does appear that the two are consistent insofar as rarely reporting links that Google is already aware are no longer in the index. We randomly selected hundreds of URLs which were "no longer found" according to our test to determine if Googlebot still had old versions cached and, uniformly, that was the case. While we can't be certain that it shows a complete set of Google's link index relative to your site, we can be confident that Google tends to show only results that are in accord with their latest data.
GSC Section 5: Search Analytics
Search Analytics is probably the most important and heavily utilized feature within Google Search Console, as it gives us some insight into the data lost with Google's "Not Provided" updates to Google Analytics. Many have rightfully questioned the accuracy of the data, so we decided to take a closer look.
Experimental analysis
The Search Analytics section gave us a unique opportunity to utilize an experimental design to determine the reliability of the data. Unlike some of the other metrics we tested, we could control reality by delivering clicks under certain circumstances to individual pages on a site. We developed a study that worked something like this:
- Create a series of nonsensical text pages.
- Link to them from internal sources to encourage indexation.
- Use volunteers to perform searches for the nonsensical terms, which inevitably reveal the exact-match nonsensical content we created.
- Vary the circumstances under which those volunteers search to determine if GSC tracks clicks and impressions only in certain environments.
- Use volunteers to click on those results.
- Record their actions.
- Compare to the data provided by GSC.
We decided to check 5 different environments for their reliability:
- User performs search logged into Google in Chrome
- User performs search logged out, incognito in Chrome
- User performs search from mobile
- User performs search logged out in Firefox
- User performs the same search 5 times over the course of a day
We hoped these variants would answer specific questions about the methods Google used to collect data for GSC. We were sorely and uniformly disappointed.
Experimental results
Method | Delivered | GSC Impressions | GSC Clicks |
---|---|---|---|
Logged In Chrome | 11 | 0 | 0 |
Incognito | 11 | 0 | 0 |
Mobile | 11 | 0 | 0 |
Logged Out Firefox | 11 | 0 | 0 |
5 Searches Each | 40 | 2 | 0 |
GSC recorded only 2 impressions out of 84, and absolutely 0 clicks. Given these results, I was immediately concerned about the experimental design. Perhaps Google wasn't recording data for these pages? Perhaps we didn't hit a minimum number necessary for recording data, only barely eclipsing that in the last study of 5 searches per person?
Unfortunately, neither of those explanations made much sense. In fact, several of the test pages picked up impressions by the hundreds for bizarre, low-ranking keywords that just happened to occur at random in the nonsensical tests. Moreover, many pages on the site recorded very low impressions and clicks, and when compared with Google Analytics data, did indeed have very few clicks. It is quite evident that GSC cannot be relied upon, regardless of user circumstance, for lightly searched terms. It is, by this account, not externally valid — that is to say, impressions and clicks in GSC do not reliably reflect impressions and clicks performed on Google.
As you can imagine, I was not satisfied with this result. Perhaps the experimental design had some unforeseen limitations which a standard comparative analysis would uncover.
Comparative analysis
The next step I undertook was comparing GSC data to other sources to see if we could find some relationship between the data presented and secondary measurements which might shed light on why the initial GSC experiment had reflected so poorly on the quality of data. The most straightforward comparison was that of GSC to Google Analytics. In theory, GSC's reporting of clicks should mirror Google Analytics's recording of organic clicks from Google, if not identically, at least proportionally. Because of concerns related to the scale of the experimental project, I decided to first try a set of larger sites.
Unfortunately, the results were wildly different. The first example site received around 6,000 clicks per day from Google Organic Search according to GA. Dozens of pages with hundreds of organic clicks per month, according to GA, received 0 clicks according to GSC. But, in this case, I was able to uncover a culprit, and it has to do with the way clicks are tracked.
GSC tracks a click based on the URL in the search results (let's say you click on /pageA.html). However, let's assume that /pageA.html redirects to /pagea.html because you were smart and decided to fix the casing issue discussed at the top of the page. If Googlebot hasn't picked up that fix, then Google Search will still have the old URL, but the click will be recorded in Google Analytics on the corrected URL, since that is the page where GA's code fires. It just so happened that enough cleanup had taken place recently on the first site I tested that GA and GSC had a correlation coefficient of just .52!
So, I went in search of other properties that might provide a clearer picture. After analyzing several properties without similar problems as the first, we identified a range of approximately .94 to .99 correlation between GSC and Google Analytics reporting on organic landing pages. This seems pretty strong.
Finally, we did one more type of comparative analytics to determine the trustworthiness of GSC's ranking data. In general, the number of clicks received by a site should be a function of the number of impressions it received and at what position in the SERP. While this is obviously an incomplete view of all the factors, it seems fair to say that we could compare the quality of two ranking sets if we know the number of impressions and the number of clicks. In theory, the rank tracking method which better predicts the clicks given the impressions is the better of the two.
Call me unsurprised, but this wasn't even close. Standard rank tracking methods performed far better at predicting the actual number of clicks than the rank as presented in Google Search Console. We know that GSC's rank data is an average position which almost certainly presents a false picture. There are many scenarios where this is true, but let me just explain one. Imagine you add new content and your keyword starts at position 80, then moves to 70, then 60, and eventually to #1. Now, imagine you create a different piece of content and it sits at position 40, never wavering. GSC will report both as having an average position of 40. The first, though, will receive considerable traffic for the time that it is in position 1, and the latter will never receive any. GSC's averaging method based on impression data obscures the underlying features too much to provide relevant projections. Until something changes explicitly in Google's method for collecting rank data for GSC, it will not be sufficient for getting at the truth of your site's current position.
Reconciliation
So, how do we reconcile the experimental results with the comparative results, both the positives and negatives of GSC Search Analytics? Well, I think there are a couple of clear takeaways.
- Impression data is misleading at best, and simply false at worst: We can be certain that all impressions are not captured and are not accurately reflected in the GSC data.
- Click data is proportionally accurate: Clicks can be trusted as a proportional metric (ie: correlates with reality) but not as a specific data point.
- Click data is useful for telling you what URLs rank, but not what pages they actually land on.
Understanding this reconciliation can be quite valuable. For example, if you find your click data in GSC is not proportional to your Google Analytics data, there is a high probability that your site is utilizing redirects in a way that Googlebot has not yet discovered or applied. This could be indicative of an underlying problem which needs to be addressed.
Final thoughts
Google Search Console provides a great deal of invaluable data which smart webmasters rely upon to make data-driven marketing decisions. However, we should remain skeptical of this data, like any data source, and continue to test it for both internal and external validity. We should also pay careful attention to the appropriate manners in which we use the data, so as not to draw conclusions that are unsafe or unreliable where the data is weak. Perhaps most importantly: verify, verify, verify. If you have the means, use different tools and services to verify the data you find in Google Search Console, ensuring you and your team are working with reliable data. Also, there are lots of folks to thank here -Michael Cottam, Everett Sizemore, Marshall Simmonds, David Sottimano, Britney Muller, Rand Fishkin, Dr. Pete and so many more. If I forgot you, let me know!
Sign up for The Moz Top 10, a semimonthly mailer updating you on the top ten hottest pieces of SEO news, tips, and rad links uncovered by the Moz team. Think of it as your exclusive digest of stuff you don't have time to hunt down but want to read!
Monday, January 30, 2017
A Brief History of Productivity: How Getting Stuff Done Became an Industry
Anyone who’s ever been a teenager is likely familiar with the question, "Why aren’t you doing something productive?” If only I knew, as an angsty 15-year-old, what I know after conducting the research for this article. If only I could respond to my parents with the brilliant retort, "You know, the idea of productivity actually dates back to before the 1800s." If only I could ask, "Do you mean 'productive' in an economic or modern context?"
Back then, I would have been sent to my room for "acting smart." But today, I'm a nerdy adult who is curious to know where today's widespread fascination with productivity comes from. There are endless tools and apps that help us get more done -- but where did they begin?
If you ask me, productivity has become a booming business. And it's not just my not-so-humble opinion -- numbers and history support it. Let's step back in time, and find out how we got here, and how getting stuff done became an industry.
What Is Productivity?
The Economic Context
Dictionary.com defines productivity as “the quality, state, or fact of being able to generate, create, enhance, or bring forth goods and services.” In an economic context, the meaning is similar -- it’s essentially a measure of the output of goods and services available for monetary exchange.
How we tend to view productivity today is a bit different. While it remains a measure of getting stuff done, it seems like it’s gone a bit off the rails. It’s not just a measure of output anymore -- it’s the idea of squeezing every bit of output that we can from a single day. It’s about getting more done in shrinking amounts of time.
It’s a fundamental concept that seems to exist at every level, including a federal one -- the Brookings Institution reports that even the U.S. government, for its part, “is doing more with less” by trying to implement more programs with a decreasing number of experts on the payroll.
The Modern Context
And it’s not just the government. Many employers -- and employees -- are trying to emulate this approach. For example, CBRE Americas CEO Jim Wilson told Forbes, “Our clients are focused on doing more and producing more with less. Everybody's focused on what they can do to boost productivity within the context of the workplace.”
It makes sense that someone would view that widespread perspective as an opportunity. There was an unmet need for tools and resources that would solve the omnipresent never-enough-hours-in-the-day problem. And so it was monetized to the point where, today, we have things like $25 notebooks -- the Bullet Journal, to be precise -- and countless apps that promise to help us accomplish something at any time of day.
But how did we get here? How did the idea of getting stuff done become an industry?
A Brief History of Productivity
Pre-1800s
Productivity and Agriculture
In his article “The Wealth Of Nations Part 2 -- The History Of Productivity,” investment strategist Bill Greiner does an excellent job of examining this concept on a purely economic level. In its earliest days, productivity was largely limited to agriculture -- that is, the production and consumption of food. Throughout the world around that time, rural populations vastly outnumbered those in urban areas, suggesting that fewer people were dedicated to non-agricultural industry.
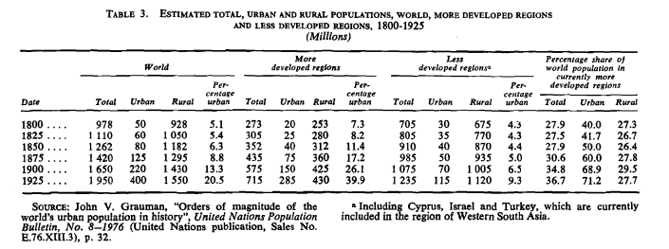
On top of that, prior to the 1800s, food preservation was, at most, archaic. After all, refrigeration wasn’t really available until 1834, which meant that crops had to be consumed fast, before they spoiled. There was little room for surplus, and the focus was mainly on survival. The idea of “getting stuff done” didn’t really exist yet, suppressing the idea of productivity.
The Birth of the To-Do List
It was shortly before the 19th century that to-do lists began to surface, as well. In 1791, Benjamin Franklin recorded what was one of the earliest-known forms of it, mostly with the intention of contributing something of value to society each day -- the list opened with the question, “What good shall I do this day?”
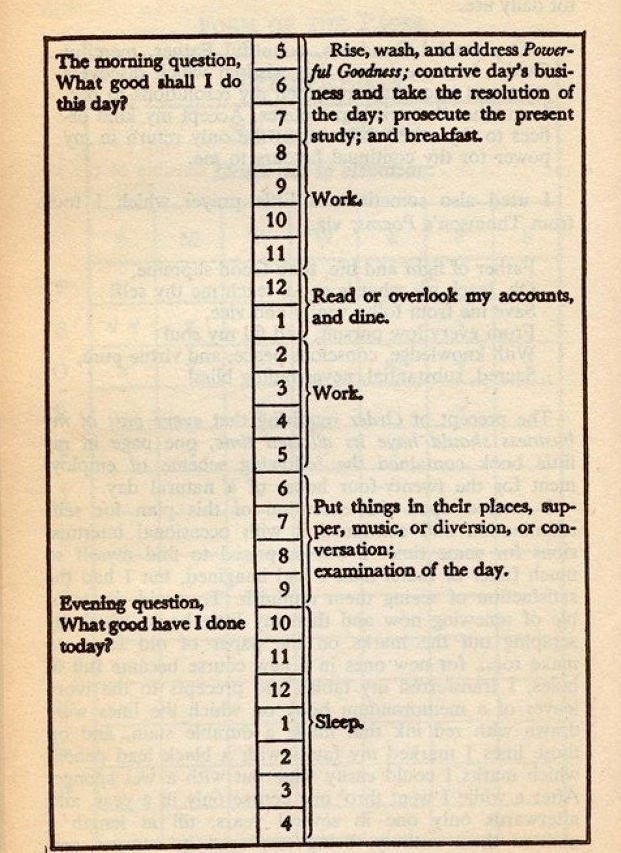
The items on Franklin’s list seemed to indicate a shift in focus from survival to completing daily tasks -- things like “dine,” “overlook my accounts,” and “work.” It was almost a precursor to the U.S. Industrial Revolution, which is estimated to have begun within the first two decades of the nineteenth century. The New York Stock & Exchange Board was officially established in 1817, for example, signaling big changes to the idea of trade -- society was drifting away from the singular goal of survival, to broader aspirations of monetization, convenience, and scale.
1790 - 1914
The Industrial Revolution actually began in Great Britain in the mid-1700s, and began to show signs of existence in the U.S. in 1794, with the invention of the cotton gin -- which mechanically removed the seeds from cotton plants. It increased the rate of production so much that cotton eventually became a leading U.S. export and “vastly increased the wealth of this country," writes Joseph Wickham Roe.
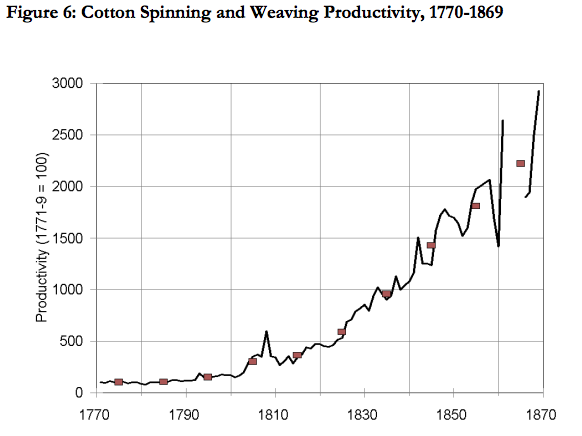
It was one of the first steps in a societal step toward automation -- to require less human labor, which often slowed down production and resulted in smaller output. Notice in the table below that, beginning in 1880, machinery added the greatest value to the U.S. economy. So from the invention of the cotton gin to the 1913 unveiling of Ford’s inaugural assembly line (note that “automotive” was added to the table below in 1920), there was a common goal among the many advances of the Industrial Revolution: To produce more in -- you guessed it -- less time.
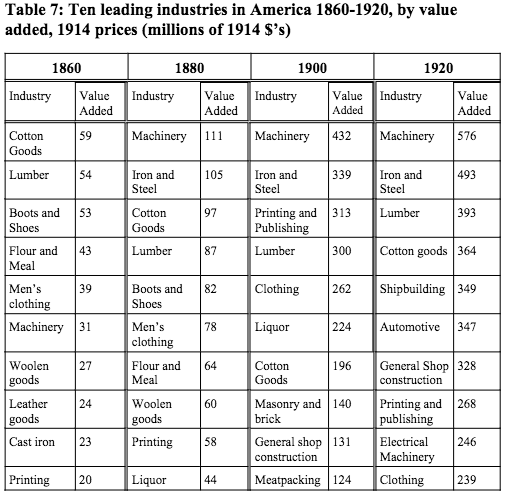
1914 - 1970s
Pre-War Production
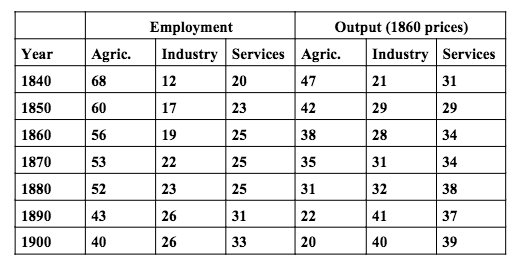
Advances in technology -- and the resulting higher rate of production -- meant more employment was becoming available in industrial sectors, reducing the agricultural workforce. But people may have also become busier, leading to the invention and sale of consumable scheduling tools, like paper day planners.
According to the Boston Globe, the rising popularity of daily diaries coincided with industrial progression, with one of the earliest known to-do lists available for purchase -- the Wanamaker Diary -- debuting in the 1900s. Created by department store owner John Wanamaker, the planner’s pages were interspersed with print ads for the store’s catalogue, achieving two newly commercial goals: Helping an increasingly busier population plan its days, as well as advertising the goods that would help to make life easier.
.jpg?t=1485761733484&width=371&name=Wanamaker_Diary_TP2%20(1).jpg)
World War I
But there was a disruption to productivity in the 1900s, when the U.S. entered World War I, from April 1917 to the war’s end in November 1918. Between 1918 and at least 1920 both industrial production and the labor force shrank, setting the tone for several years of economic instability. The stock market grew quickly after the war, only to crash in 1929 and lead to the 10-year Great Depression. Suddenly, the focus was on survival again, especially with the U.S. entrance into World War II in 1941.
But look closely at the above chart. After 1939, the U.S. GDP actually grew. That’s because there was a revitalized need for production, mostly of war materials. On top of that, the World War II era saw the introduction of women into the workforce in large numbers -- in some nations, women comprised 80% of the total addition to the workforce during the war.
World War II and the Evolving Workforce
The growing presence of women in the workforce had major implications for the way productivity is thought of today. Starting no later than 1948 -- three years after World War II’s end -- the number of women in the workforce only continued to grow, according to the U.S. Department of Labor.
That suggests larger numbers of women were stepping away from full-time domestic roles, but many still had certain demands at home -- by 1975, for example, mothers of children under 18 made up nearly half of the workforce. That created a newly unmet need for convenience -- a way to fulfill these demands at work and at home.
Once again, a growing percentage of the population was strapped for time, but had increasing responsibilities. That created a new opportunity for certain industries to present new solutions to what was a nearly 200-year-old problem, but had been reframed for a modern context. And it began with food production.
1970s - 1990s
The 1970s and the Food Industry
With more people -- men and women -- spending less time at home, there was a greater need for convenience. More time was spent commuting and working, and less time was spent preparing meals, for example.
The food industry, therefore, was one of the first to respond in kind. It recognized that the time available to everyone for certain household chores was beginning to diminish, and began to offer solutions that helped people -- say it with us -- accomplish more in fewer hours.
Those solutions actually began with packaged foods like cake mixes and canned goods that dated back to the 1950s, when TV dinners also hit the market -- 17 years later, microwave ovens became available for about $500 each.
But the 1970s saw an uptick in fast food consumption, with Americans spending roughly $6 billion on it at the start of the decade. As Eric Schlosser writes in Fast Food Nation, “A nation’s diet can be more revealing than its art or literature.” This growing availability and consumption of prepared food revealed that we were becoming obsessed with maximizing our time -- and with, in a word, productivity.
The Growth of Time-Saving Technology
Technology became a bigger part of the picture, too. With the invention of the personal computer in the 1970s and the World Wide Web in the 1980s, productivity solutions were becoming more digital. Microsoft, founded in 1975, was one of the first to offer them, with a suite of programs released in the late 1990s to help people stay organized, and integrate their to-do lists with an increasingly online presence.
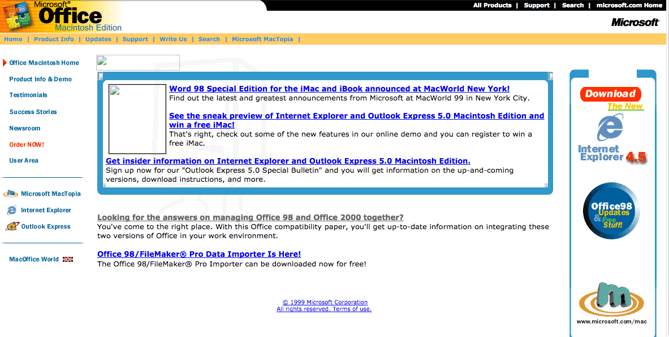
It was preceded by a 1992 version of a smartphone called Simon, which included portable scheduling features. That introduced the idea of being able to remotely book meetings and manage a calendar, saving time that would have been spent on such tasks after returning to one’s desk. It paved the way for calendar-ready PDAs, or personal digital assistants, which became available in the late 1990s.
By then, the idea of productivity was no longer on the brink of becoming an industry -- it was an industry. It would simply become a bigger one in the decades to follow.
The Early 2000s
The Modern To-Do List
Once digital productivity tools became available in the 1990s, the release of new and improved technologies came at a remarkable rate -- especially when compared to the pace of developments in preceding centuries.
In addition to Microsoft, Google is credited as becoming a leader in this space. By the end of 2000, it won two Webby Awards and was cited by PC Magazine for its “uncanny knack for returning extremely relevant results." It was yet another form of time-saving technology, by helping people find the information they were seeking in a way that was more seamless than, say, using a library card catalog.
In April 2006, Google Calendar was unveiled, becoming one of the first technologies that allowed users to share their schedules with others, helping to mitigate the time-consuming exchanges often required of setting up meetings. It wasn’t long before Google also released Google Apps for Your Domain that summer, providing businesses with an all-in-one solution -- email, voicemail, calendars, and web development tools, among others.
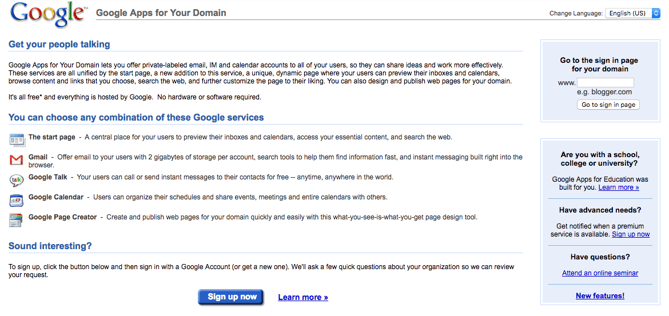
During the first 10 years of the century, Apple was experiencing a brand revitalization. The first iPod was released in 2001, followed by the MacBook Pro in 2006 and the iPhone in January 2007 -- all of which would have huge implications for the widespread idea of productivity.
2008 - Present
Search Engines That Talk -- and Listen
When the iPhone 4S was released in 2011, it came equipped with Siri, “an intelligent assistant that helps you get things done just by asking.” Google had already implemented voice search technology in 2008, but it didn’t garner quite as much public attention -- most likely because it required a separate app download. Siri, conversely, was already installed in the Apple mobile hardware, and users only had to push the iPhone’s home button and ask a question conversationally.
But both offered further time-saving solutions. To hear weather and sports scores, for examples, users no longer had to open a separate app, wait for a televised report, or type in searches. All they had to do was ask.
By 2014, voice search had become commonplace, with multiple brands -- including Microsoft and Amazon -- offering their own technologies. Here’s how its major pillars look today:
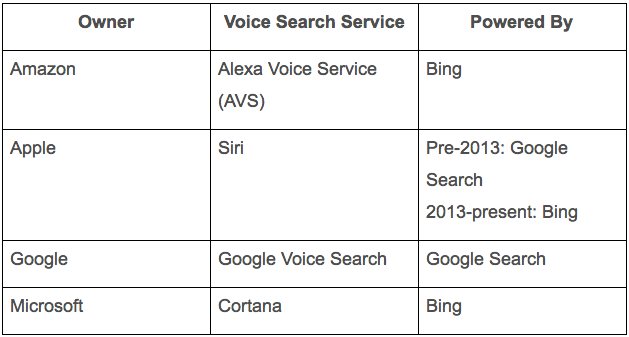
The Latest Generation of Personal Digital Assistants
With the 2014 debut of Amazon Echo, voice activation wasn’t just about searching anymore. It was about full-blown artificial intelligence that could integrate with our day-to-day lives. It was starting to converge with the Internet of Things -- the technology that allowed things in the home, for example, to be controlled digitally and remotely -- and continued to replace manual, human steps with intelligent machine operation. We were busier than ever, with some reporting 18-hour workdays and, therefore, diminishing time to get anything done outside of our employment.
Here was the latest solution, at least for those who could afford the technology. Users didn’t have to manually look things up, turn on the news, or write down to-do and shopping lists. They could ask a machine to do it with a command as simple as, “Alexa, order more dog food.”
Of course, competition would eventually enter the picture and Amazon would no longer stand alone in the personal assistant technology space. It made sense that Google -- who had long since established itself as a leader in the productivity industry -- would enter the market with Google Home, released in 2016, and offering much of the same convenience as the Echo.
Of course, neither one has the same exact capabilities as the other -- yet. But let’s pause here, and reflect on how far we’ve come.
Where We Are Now...and Beyond
We started this journey in the 1700s with Benjamin Franklin’s to-do list. Now, here we are, over two centuries later, with intelligent machines making those lists and managing our lives for us.
Have a look at the total assets of some leaders in this space (as of the writing of this post, in USD):
- Amazon: $6,700,000,000
- Apple: $293,280,000,000
- Google: $159,948,000,000
- Microsoft: $181,870,000,000
Over time -- hundreds of years, in fact -- technology has made things more convenient for us. But as the above list shows, it’s also earned a lot of money for a lot of people. And those figures leave little doubt that, today, productivity is an industry, and a booming one at that.
How do you view productivity today, and what’s your approach to it? Let us know in the comments.
Fact or Fiction: The SEO Edition [Quiz]
Just when you thought you were up-to-date on the latest SEO best practices, an algorithm change or a new expert post has you questioning yourself. Sound familiar?
It's true, the world of SEO is spinning faster than ever, and for every new strategy, an old one becomes obsolete. As inbound marketers, it's our job to stay informed of these changes, while continuously questioning the status quo.
Today, successful inbound marketers turn their attention to a long overdue focus on high-quality content. Marketers and SEO agencies worldwide have halted their obsession with link building and keywords and re-prioritized for this specifically.
Is your team still focusing on keywords rather than topic clusters? How about meta descriptions - how has Google been treating them differently as of late? See how you and your team fare in the new world of search engine optimization by taking this 10-question quiz.